There has been a recent increase in discussions around biases resulting from the use of AI and machine learning, what is being referred to as algorithmic bias. For example, the use by Amazon of a recruitment tool favouring male applicants and claims against Google that results from the search engine favour certain political parties.
As the use of AI and machine learning becomes more commonplace in daily life, in areas such as criminal justice, education, health and welfare, and recruitment, then it becomes more critical to be aware of such issues and attempt to mitigate them. In this post we review some of the current discussions around bias in AI and machine learning.
Uses of AI and machine learning
AI refers to technologies that enable computers to learn, reason and assist in decision-making; captured using hand-crafted rules or, more commonly, using machine learning (programs that automatically improve their outputs with experience). Supervised machine learning methods (e.g., classification) use labelled data as training examples to make future predictions.
Labels typically take the form of categories that form the goal of the prediction, e.g. ‘high risk’ or ‘low risk’. Unsupervised machine learning methods (e.g., clustering), on the other hand, makes use of unlabelled data and attempt to identify structures or groups within the data.
A 2016 UK Government report on the opportunities and implications of using AI highlighted a range of use cases for technology, such as the personalisation of services, identifying environmental and socio-economic trends, the optimisation of storage and distribution networks, and the management of healthcare.
The focus of the 2018 Microsoft Future Decoded conference and their associated report “Maximising the AI opportunity” was also the use of AI as a vital element in the digital transformation of businesses. Companies from all sectors are investing in AI and making use of machine learning to assist people with (or in some cases entirely automate) business decision making and it is clear that numerous benefits of such investment abound (see, for example, the UK Government report).
Biases in AI and machine learning
Perhaps less well understood (but increasingly the attention of media) is that AI and machine learning can also encapsulate and perpetuate biases in various forms, such as stereotypes. The notion of bias captures situations in which an inclination or prejudice towards one person or group is exhibited and could be considered unfair. For example, political bias might be presenting an unbalanced view of political parties; gender and racial bias might show itself as favouring one group over another.
That computational systems, such as AI and machine learning, are biased is not new and issues surrounding this have been raised for decades. For example, academics Batya Friedman and Helen Nissenabaum wrote about bias and gave a number of examples in their 1996 article “Bias in Computer Systems.” However, what has changed since then is the rapid rise and availability of data, new developments in AI and machine learning, and their wider use in decision making across all areas of business and daily life.
As a result concerns have been raised: “After all, it’s an old saying in computer science: garbage in, garbage out. And the reality is that our biases (political, racial and gendered) show in the data that we feed to our AI algorithms” (Forbes, 2018) and “Unless used very carefully, data science can actually perpetuate and reinforce bias” (John Kelleher and Brendan Tierney in their book on Data Science).
Examples of bias
But what do these biases look like and can they really affect people’s lives? Researchers from the Oxford Internet Institute and the Alan Turing Institute provide various examples of algorithmic bias in their article on the ethics of algorithms. These include systems for profiling and classification, making recommendations, personalisation and filtering, data mining, and decision making and we provide three examples next.
In the case of the AI tool being tested by Amazon for recruitment purposes, this was being used to help sift through large volumes of CVs to find potential promising applicants. However, the system learned to prefer male candidates over female candidates, therefore exhibiting gender bias. Upon investigation the problem was found to be the historical data used to train the models: over a 10-year period the majority of the data in the form of past CVs came from men; therefore, the system biased itself towards preferring male applicants.
Another widely cited example is the use of predictive analytics within the legal system and law enforcement. For example, AI and machine learning is increasingly being used to steer decisions around which neighbourhoods or areas police should focus their attention on (known as ‘predictive policing’). However, as this report on Durham Constabulary highlights, the algorithms can end up discriminating certain groups, such as the poor. In addition, by focusing on certain groups, the systems can perpetuate biases as target groups become increasingly profiled, through which more data is gathered that subsequently reinforces future predictions, and so on.
Predicting crime hotspots is one thing, but perhaps of more concern is the profiling of individuals, for example as to whether they are likely to commit crimes or pose risk to the public. A 2016 report by ProPublica found that software used by US courts for assessing the risk of people re-offending (called COMPAS) was biased against black offenders. They were indicated as ‘high risk’ by the software, but subsequently never charged with another crime.
Finally, an example that we all encounter daily - biases on the web and in particular within search engines, such as Google. For example, reports suggest that Google’s rankings are not objective, but favour certain topics, groups of people or products. For example, in the US Google has been accused of favouring Democrats by showing more results from this political party in the page of results.
Another example is the promotion of Google’s own shopping comparison sites at the top of search results ahead of its competitors, which resulted in a £2.1 billion fine from the European Commission. However, in the case of web search biases may not be intentional, but the result of a highly complex socio-technical system in which people and their interactions with technology shape the outcomes of search. Perhaps of more concern is the lack of awareness of biases by users of web search and the lack of digital literacy skills needed to ensure people evaluate and question what they find (e.g., ‘real’ vs. ‘fake’ news).
Actions being taken
A reaction towards bias in AI and machine learning could be one of distrust and to not use such methods in decision making processes. However, despite the hype the reality is that biases exist in any form of decision making, with or without technology: humans are inherently biased and this can manifest it within stereotypes and the decisions we make, e.g. we prefer certain candidates in an interview because they are more like us (i.e., familiarity bias).
As a 2017 article by McKinsey & Company on algorithmic bias highlights: “Myths aside, artificial intelligence is as prone to bias as the human kind. The good news is that the biases in algorithms can also be diagnosed and treated.” In fact a 2018 Harvard Business Review article on decision making states that the algorithms are in practice less biased and more accurate at making decisions than the humans they are replacing (or assisting).
Rather than not use technology we need to understand its strengths and weaknesses and control its use. In the case of AI and machine learning we need to understand where biases can be found and
mitigate their effects where possible. This has led to calls for accountability, especially towards those who create and manage the technologies and transparency.
This latter point is something highlighted within the 2019 report on Business Intelligence Trends from Tableau: “Many machine learning applications don’t currently have a way to “look under the hood” to understand the algorithms or logic behind decisions and recommendations, so organisations piloting AI programs are rightfully concerned about widespread adoption.”
Providing adequate governance and consideration of ethics is also seen as a key factor to controlling the future use of AI and machine learning and forms a part of the General Data Protection Regulation (GDPR).
Accenture addresses this in their Universal Principles of Data Ethics report, where they state that “governance practices should be robust, known to all team members and reviewed regularly.” The Data Ethics Framework from the UK Government Digital Services provides organisations with clear principles to how data should be used, and despite being focused on the public sector has clear relevance to any business utilising data, AI and machine learning technologies for decision making.
The major IT vendors are also making their contributions by releasing tools to help AI developers explore and counteract biases in their datasets and algorithms. For example, the Google What-If tool helps users to analyse machine learning models in response to changes in parameters and data to help identify bias. Similarly, IBM has introduced their AI Fairness 360 toolkit which provides a comprehensive open-source toolkit of metrics to check for unwanted biases in datasets and machine learning models.
Finally, this report by McKinsey & Company on “Controlling machine-learning algorithms and their biases” identifies at least the following three ways that AI and machine learning biases can be reduced from the perspective of different groups of people:
How Machine Learning Biases Can Be Reduced
- Users of machine learning need to understand the strengths and weaknesses of algorithms and how they can be influenced and shaped by biases.
- Data scientists developing the algorithms need to select balanced and unbiased data samples and take steps to ensure algorithmic biases are minimised (e.g., use of cross-validation to avoid overfitting and methods to deal with imbalanced classes).
- Executives and managers should know when (and when not) to use machine learning algorithms in the business.
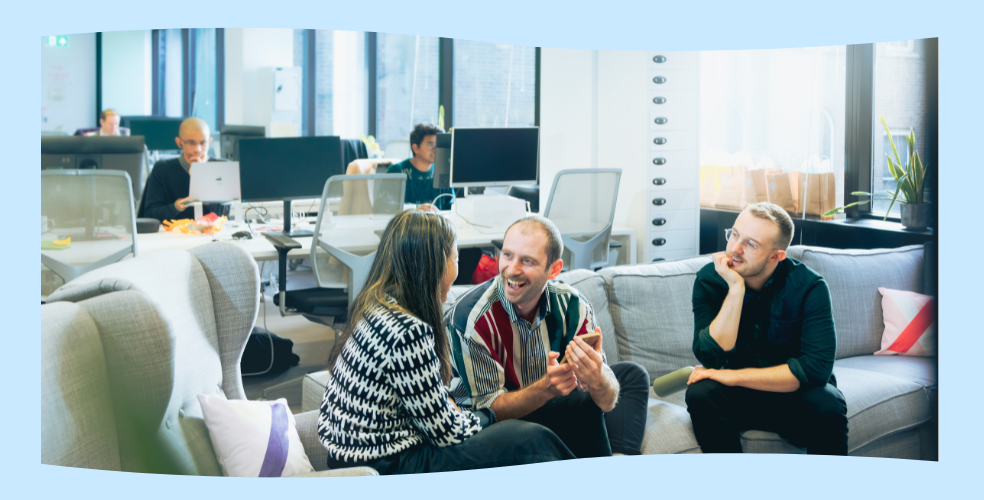
Implementing a global people analytics system for a leading bank
How we delivered a scalable HR analytics platform for one of the world’s biggest banks to meet its people's needs
Read moreOur recent insights
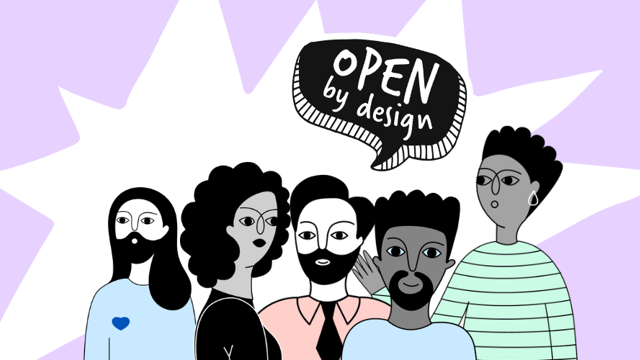
Local Digital: uniting for better public services
Bringing together ambitious changemakers to share radically new, standards-based models and tools for local public services
Read more
A design system for transformational change
We've partnered with Hackney Council on several projects from housing to employment, always making sure services are understood, fair, accessible and beneficial to residents, supported by flexible modern technology.
Read more
How technology can improve mental health patient care
Automation technology can improve waiting times, patient communications, and outcomes in Mental Health Trusts.
Read more