On their journey through the data lifecycle, the discussion touches on fairer data collection, how to involve voices from across the organisation, and establishing a culture of continuous improvement.
In this episode, we cover advice for businesses of all sizes and sectors. With just a little self-reflection, organisations can often steer clear of the worst-case scenarios, so switch-on and tune-in to episode two of Discussions on Data Ethics.
You can catch up on the first episode in the series here or
Nathan Makalena - NM
Paul Clough - PC
Lucy Knight - LK
NM - Well, I think it's worth talking over the lifecycle in a bit more detail. We go from collection, to storage, to usage, to sharing, to destruction. I guess, broadly, which of those areas do you think are the most mature in terms of data ethics? And which do you think, are the ones that people maybe haven't thought as much about?
LK - Most mature, from my experience, is probably going to be storage and sharing, there's a lot of awareness around what is okay, what is not what tools exist.
Least mature, I'd say collection. Right at the very beginning people are not necessarily asking themselves, ‘Do I need this data? Do I need all of this data?’ And step before that, which is, ‘What problem am I solving? What question am I answering with this project, with this analysis?’ And therefore, ‘What would make sense? If I had an answer, what would it look like?’ These are simple little questions, that you can bookend your whole process with to examine really, what, what even am I doing here?
When we do the Introduction to Data ethics, which is one of the modules on the data ethics professional course, and we go through the canvas, people sometimes ask me, which is your favourite, which one would you say is most important? I always say, it's the one that says purpose.
Why are you doing this? If you can’t answer that question, you have bigger problems than GDPR. ‘What are you aiming to do? What problem is that you're supposed to be solving? If you can't answer that question. You need to stop and think.
PC - I'd agree. And I think, for me, storage is mature. There's lots of principles around things like data protection policies. A lot of GDPR does focus around some of those. And I think a lot of companies do that really well.
To be fair, as well as the collection, I'd say the usage bit as well. There's still some unknowns around that, particularly as we go into these algorithms, we're going to use machine learning AI and so on. And I think people are still not aware that, whatever you put into the algorithms, into the machine learning, is going to perpetuate.
If you've got any biases, if you've got poor data quality, it doesn't magically make anything better. What it does is actually perpetuate some of those issues and bring them to the surface. And I think a lot of people I encounter don't fully appreciate that, they kind of think that that black box can magically solve things.
And I think it's about that kind of raising awareness. There also needs to be some kind of, I think, tools and procedures to help people as well.
But I also agreed on the collection side, I've seen people who it's like, ‘Oh, it doesn't matter how we collect the data, it’s going to be cleaned up later down the line.’ That's completely the wrong way to think about it. Rubbish in rubbish out. Whatever you do at the beginning will have an impact downstream. And unless you actually actively go to kind of improve the data quality and so on, it just goes right the way through the whole process.
And whatever you use it for, poor data quality comes out on dashboards, in Excel spreadsheets, and so on in the numbers. And then that affects the decisions that you're making. So I think again, it's quite hard to say which is the most important, because in one sense, they're all important.
LK - Absolutely. I'm just nodding along with everything Paul’s saying. But I mean, he's absolutely right, then we can talk about which is the most important and the answer is all of them.
We can talk about which ones we think people we wish people would focus on more. And for me, collection first. And then I think you're absolutely right, that usage and people thinking about the ways in which they deploy whatever toolset, whatever platform they've chosen.
They're not absolved of responsibility, once they plug it into the black box. There are not unicorns and pixies in there. The machine is not infallible. You still have to have that human oversight of how that model is working. You need to be able to open the lid to have a look and not just blindly trust the machine.
PC - I think what's the challenge in one sense is that that's actually true of any kind of decision making system. So although we focus very much on computational and AI and so on. Actually, you want to be able to open the box and explain decisions made by people.
What's happened, I think with a lot of the AI, is bring things out and into the forefront. A lot of media have, rightly so, highlighted some of the issues. But to me, actually, what's good about it is that in any human system, in decision making, there are biases, there are issues and a lot of them are black boxes. And actually, we can use AI machine learning to help us understand how those human black boxes make decisions as well. So actually, I think it's kind of like bigger than just the kind of thinking about machines, we do focus on the machine, particularly around AI ethics. But I think, is a broader issue that we need to be considering.
LK - I’m fond of saying, you know, it's people all the way down. When you look at these processes, people choose the problem that needs solving, people explain or define why they think it's a problem, people decide what a solution looks like, what a good outcome looks like, people decide what data supports that, people decide what data goes into the analysis, and people decide whether or not they're going to use the outputs. You know, it's people, people, people all the way through, we use the tools, but the humans are normally still in charge.
PC - And so I think, what I've learned over the years now looking at data ethics, it is especially hard to make a change. Ethical change is a whole shift in culture. And actually, that can be quite difficult for many people and organisations as well.
But actually, it's the only way that you will ever mature. And I think actually, for a company that wants to undergo digital transformation, that process is not just ‘oh, I need to move stuff to the cloud, I need to use this technology, that technology and so on.’ It's actually about a whole culture shift. And the ethics is a really important part of that.
LK - It's massive. I’m thinking about the data maturity model and tool (put out by data orchard), which is really excellent. And it works for organisations at a much smaller scale as well.
There are seven pillars, and two of them: one is culture, one's leadership. Yes, there's tools. Yes, there's techniques. Yes, there's, you know, how good your people are at wrangling spreadsheets. But there is very much this: ‘if you do all of this work, how often does the leadership look at it?’ Do they even use it? Or are you just howling into the void?
You know, around the culture, how often do people discuss what the data says. Do you know what is out there that's available to you to use? Who do you talk to? Who in your team do you feel has the expertise.
And across those pillars, no one is more important than the other, they all come together, and combine into your data maturity score. The organisations that are really strong in all areas, you will notice, are really strong when it comes to leadership and culture. You actually cannot call yourself strong in the other areas, if you don't have those. It simply can't happen.
PC - If you're in an environment where it hasn't worked out, there's a problem with the algorithm, and we need to fix it. You know, actually, that's what you need to get to. That's greater maturity.
And I think that's where the whole accountability comes in. You can't just wash your hands of it. Somebody needs to be held responsible, but don't point fingers in a sense. Responsibility has got to be from the top and working its way down. Which is actually why I think data ethics needs to be taught, not just the data worker level, but right the way up to the CEO.
LK - Absolutely. Absolutely agree. Every every possible level. And also that culture of safety. We talk about accountability, that culture where if anybody spots something wrong, they feel safe to point it out. Yeah, that there is a culture, there is an environment in that organisation where somebody can say, ‘I've just found this, I'm quite concerned, I think we should look at that, again’, and not be shouted down and not be penalised. That's a very rare and precious thing. And we should be encouraging that sort of attitude towards this. You can't fix it. If no one is, you know, everyone's afraid to tell you it's broken,
PC - I guess thinking through that is the topic of diversity as well. I also do some work for Digital Catapult, part of the MI,machine intelligence garage. They have an ethics advisory group for AI startups. And in their framework, one of the things that we often talk about is the kind of the business, the organisation as a whole.
And something we include is like, how diverse is the organisation? And it's mainly for what you're suggesting there Lucy, that by bringing in diverse people, you get a different set of perspectives, but also you, you're far more able to see some of those issues that you just wouldn't sport if you were all the same.
And I think actually, a lot of organisations struggle with this. And I've noticed over time, for me, working in a very technical, engineering-type of domain - which is always dominated by certain groups of people. Now, it's widened out a bit. Actually that's super positive, because you now find all those different perspectives. And that includes people from non technical backgrounds, who approach problems in completely different ways.
And that's so helpful and refreshing. And actually, I think it's really positive because it means if we were employing for a technical post, we could be employing arts and humanities students. Probably because they approach things in a super different way that we would never have thought of. And it comes back to thinking about why you're doing this in the first place. People with different perspective will raise things that you never even thought about,
LK - They will. They'll ask the unconsidered questions.
PC - And again. I think hopefully, what we've tried to bring out is that when you think about the data lifecycle, it's a journey. It doesn't just stop (you start there, you end there), but actually, you've got to keep going.
I think that's the challenge for a lot of organisations. Turning it into the life and the breadth, what you live by, as an organisation. Like you would with other things like data protection, other ways of working, that's part of the company culture.
NM - Yeah, I understand that as a sort of iterative process. I guess, as you go along. And you mentioned we're anti-tick box with this guide. I think instead of that we have these, ‘ask yourself questions’, getting people from around the organisation involved in these discussions so that your application of data can become more and more ethical, the more you learn.
LK - Yeah, turning it into a compliance thing is both helpful and massively unhelpful. If it's compliance, people pay attention. But also, they may feel if they tick that box, they've gone far enough. It's more about that self examination, self reflection, is this the right thing to do? Is this the right way to do it? that needs to be asked.
NM - I mean, even as more documentation is coming out about ethical decisions, it seems that organisations asking these questions of themselves are almost asking, you know, how, how far will I go? How far will I push this?
One of the areas where we see that or have seen that in the past is opt in checkboxes for data collection. Obscuring the language that is used around those sorts of agreements, when people sign-on. How much of the problem and the problems that come up in this report, are organisations getting away with it? I guess, getting away with the sort of level of education that people that are using these or ticking these boxes, maybe have around the full impact of what they're signing.
PC - I think in one sense, data ethics to me is, to go beyond getting away with it. So some of the ‘getting away with it’ is to tick the box of Legal Compliance, GDPR, and so on, which I think you have to do. And legally, you need to do that around areas like consent, data storage, some of those types of things.
But actually data ethics, to me, is about that but more. You know, wanting to be legally compliant but also doing things in an ethical way. It's not just about can we do this? But should we be doing this, and sort of reasoning through some of that.
And I think that happens right away across the board. Before you mentioned areas like consent, you know, and trying to gain consent. When we've talked before as well, there've been various attempts at how you gain consent. How do you go about gaining consent? Is it as simple as kind of an opt in opt out? I think sometimes it probably is.
LK - I feel very strongly that it should be an opt in. You know, explicit, active, informed. People that are going to opt-in, they should know that that's what they're opting in for.
I suppose my soapbox, my high horse that you'll never knock me off, is that if an organisation, if I get to their website and they want to set cookies, and they want to harvest my data, and they asked me. I will notice if they make it significantly easier to just say accept by having that button be where I'd expect to see the no or cancel button.
And for those organisations, I make a special point of scrolling down, however many pages it takes, and ticking all of the consent and all of the legitimate interest buttons that have been pre-ticked positive for me. And it's quite interesting to see with those organisations, it's very clear that their business model is not the thing I'm there for. The product is not the thing I’m there for, the product is me.
Because you can then see, when they say ‘about our data sharing…’ there will be like 57 organisations who are waiting to get ahold of my browsing data. And that is the business model.
So I think there's a little bit about educating people about what they are signing up for. That’s one thing, there's something about making that language better and clearer and more accessible. But there's also something about the organisation's themselves understanding that, if their entire business model is predicated on selling eyeballs, that this is not going to last forever. And it's not sustainable. And they need to think quite hard about whether they would rather have happy customers or happy sponsors. And can they actually do what they're doing without that dark pattern behind the scenes harvesting?
NM - Yeah, because it's not just a matter of then, I guess, signposting at each stage: ‘how our customers' data is being used, how it's being shared, how much is being captured.’
Another one of the problems we talked about is ‘minimal viable collection’. And that seems again, born out of a mindset of, I must take as much as I can, because something will be valuable to me or sponsors at some point along the journey.
LK - Yeah. GDPR expressly forbids that. You should not collect data because you think it will be useful later. That needs to be quite clearly understood. We were talking earlier, about the stages where we feel organisations are less mature. That's the exact example when we talk about collection: “I will just collect everything, because we might use it later.” I was like, no, no, you do not you cannot. What problem are you solving?
Now, if the problem you're trying to solve is, ‘how can I get maximum money from the advertisers’, then yes, that would be the correct tactic. It would still be illegal, though. So I think organisations need to be more cautious about chasing that model. I don't see it being super sustainable forever.
PC - I mean, I think consent also to me is quite a complex issue. In the sense of sometimes it's not as easy as ticking the box. I'm just thinking, for example, you go into hospital, or you’re under stressful situations, and you're having to make decisions and provide consent, it becomes quite difficult.
We were also talking about accessibility. How many of those long pages and pages of forms are actually accessible? What about people who have disabilities or people who are visually impaired? How ill a screen reader go through that? Would they spend 10 minutes listening to all of that? And then if that's not happening successfully, how on earth are they expected to give consent? They'll probably give up, just take the box. And off they go.
LK - Of course, if you're in a rush. I mean, I was trying to do something on the NHS app yesterday before I left home. And it turned out just not to be possible, because every link that I followed took me to something else that I didn't need. And in the end, I gave up. I closed it.
And that’s a mark of my existing privilege that I'm able to say, ‘I'll sort that out another time by talking to someone’. Okay, if someone was in dire need of an appointment or prescription or to talk to a professional, and didn't have the luxury of giving up? It very much depends on are you in crisis? Are you in need? Are you in a hurry? Do you have the luxury of saying this can all be sorted out if I just talk to someone, which is a very middle aged white woman point of view? Not everybody has that safety, that psychological safety and that luxury. So we do need to be thinking about that.
PC - And I think, you know, this is what the ethical perspective gives you I think. Its that we're viewing it from a different way. Have we provided the tick box that enables people to give consent? Yeah. Is it a mechanism that actually is usable? Does it serve its purpose and so on? Well, now we're not so sure. Now we're having these conversations.
And I think this is then ‘the ethical’. This is why it goes broader and greater than just ‘well, you've ticked the legal compliance. ’ I think this is what is really important about making sure you really get involved with ethics as well, because it’s much more than just compliance. We wouldn't be having these conversations and so on. And you wouldn't be thinking about these groups of people unless you were kind of taking a more kind of, I think ethical perspective to be fair, yeah.
NM - Holistic view. I guess then there's a question there about, iterative life cycles and improving things as they go on. You know, at what point does abandoning a project become the best course of action, I guess, rather than trying to dip in and change things and adjust things towards more ethical decisions?
LK - I was talking earlier about how certain business models may become less and less sustainable. I do think that unmoderated social media content as a business model is going to become less and less sustainable.
Because up to a point, the platform can say’ we're simply a platform, we're not a content creator. We simply provide a platform for other people, therefore, it's not our fault if anything bad happens.’
But then if you look at the way those recommender algorithms begin. If you signed in today to YouTube, or similar, to Tik Tok and so on. If you signed in today, anonymously, the first 100 things you would be shown would be extreme content. Quite a lot of it right wing, misogynist, violent, sexist content, because that is very popular.
When I go onto Steam, and look for what's new in the games, I'm always being shown because it is popular. It's like, that's nice. But I've already asked Steam specifically not to show me any of that content, not because it's offensive, because I simply don't like puzzle platformers. That's my preference. But it will still slide them into my discovery queue because it's popular with other people.
And this is how they then whittle down ‘what are you interested in?’ but the first thing they show you is stuff that is quite polarising. Because it’s trying to figure out, like in diagnostics, in which half of this system is the problem. ‘We'll show you one half, if you violently reject that, we'll show you the other half.’ This is a problem.
And that business model is sustainable only because you don't have to throw lots of human labour at it. We can't get away with that for much longer, because as in Molly's case, people are getting hurt.
PC - And this is this kind of, I think, the other argument from why you've got to monitor. It's fine to put an algorithm out there, but you must monitor it because it changes over time.
What happens is a lot of these algorithms are fed by humans and social interaction. Microsoft had to shut down its Tay (Chatbot) mainly because people started to feed it quite vile language. And it started to repeat that
LK - I think it took about a day and a half for Tay to go from teenage innocent to violent Nazi.
PC - Yeah, yeah. Did Microsoft ever want that intent? Of course they don't. But you have to understand that people can misuse technology and abuse it and whatever. And then we're back to the well, who's accountable, who should be shutting it down? Who picks up the pieces and so on.
And this is why I think with data ethics, to have these clear frameworks, these principles in place, is really important.
Because you asked the question, is it going to go wrong? How could it go wrong? One of the things we do at the Machine Intelligence Garage is a headlines scenario. Imagine a headline, the worst for your company, you know, which involved AI data and so on. What are you gonna do about it? Have you got the governance structure in place to deal with it? What comms are you going to give to people, when people complain, and so on? Who's going to respond? How are you going to respond? If you shut it down? Do you have a business model that can hold that? You know, what things are in play that would not get it shut down? And so on? Yeah, I think that's so important to have all this structure.
LK - Exactly, envisage that. And one thing I sometimes do with people is exactly that scenario. Imagine the worst possible outcome. Now imagine if you wanted to cause it, how would you do it?
And that gets them going, ‘Oh, right. Yes. If I did this, and I would do, oh, god. If the director did that, oh, yeah, that would do it…
And now you have the little actions, not just reacting. But actually the small things you need to be looking out for within your organisation. The ways people behave, the mistakes you might make, or your colleagues might make, that you can kind of now start heading off.
I worked with a small organisation. And I basically said, this is the contract, this is the project, this is what you want to happen. Now I want you to do some ‘disaster imagining’. Basically, if we were going to shut this down and make sure that it never happened, or happened so badly that no one will ever want to work with this again, how would you make it happen?
And they came up with three things that went straight to the top of the risk register, and one of them did include a change of upper management, and a new person coming in and going. ‘I don't understand this, I don't like it, I'm putting a hold on it.’ And that actually did happen. But because we had it on the risk register, they were able to address it.
PC - And although you might say ‘Oh, I could never predict all the things that could go wrong.’ And that's fair, you can’t.
But actually there are organisations - I'm thinking of Cardiff University - have an algorithm watch, or AI watch. Basically a register, cases where algorithms have gone wrong and cause effects. It's a catalogue, and other countries have this as well, the OECD has a register as well. And actually, it's becoming harder to say, ‘I could never imagine that would happen’, because actually, there are records that are kept. It's just you've got to go out and be aware of it. You have to be of the mindset that things can go wrong. And if you take the attitude of ‘Well, ‘I just don’t know’. I just don't think that's gonna cut it.
LK - I don't think I would want to do business with a company that went ‘Phoar, who could have seen that coming?’
PC - So, I think the whole kind of implementing data ethics is tricky in one sense, I don't think it's as simple as a checklist, even though it’d be lovely if it were.
I think tools like the ODI data Canvas are really helpful, particularly on a project basis. I think probably for me a place to start would be as an organisation, maybe think about your underlying values. Those principles by which you as an organisation are living and breathing.
So it might be things like, ‘we will manage people's data responsibly’, and then just say what that means to you. And, you know, work on these shared values as an organisation, get people from across the organisation to feed into it so that everybody buys into it.
I think that's a really good place to start because it starts to sort of set the tone of what you’re trying to achieve as an organisation. Now how we actually do that is a different thing. But for me, that would be a good starting point. You know, what is it we believe? How do we want to operate as an organisation particularly from an ethical kind of perspective?
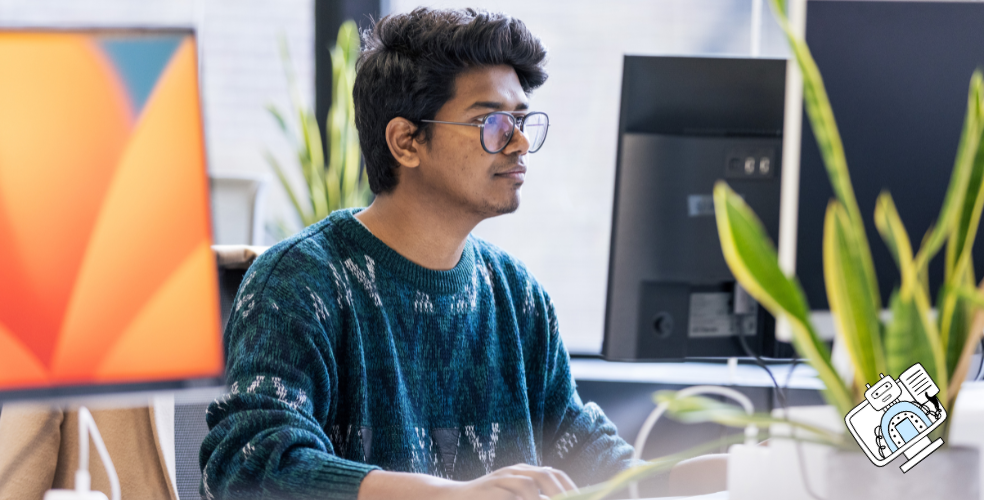
From SQL Server, to Azure, to Fabric: My Microsoft Data and Analytics journey
There is always something new in the Microsoft space, and Fabric is the latest game-changing update to impact the world of analytics.
Read moreOur recent insights
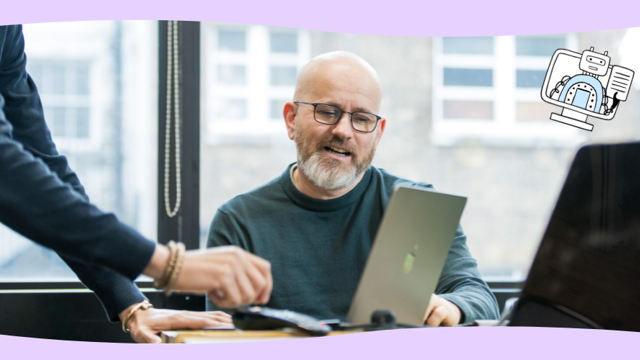
Common misunderstandings about LLMs within Data and Analytics
GenAI and LLMs have their benefits, but understanding their limitations and the importance of people is key to their success.
Read more
Shaping product and service teams
How cultivating product and service teams to support the needs of the entire product lifecycle can ensure brilliant delivery.
Read more
Building ‘The Chatbot’ - Our experience with GenAI
Learn how we harnessed to power of Generative AI to build our very own chatbot.
Read more