It’s not uncommon to come across ‘become data driven’ as an annual objective, whether at a fledgling start ups or a public sector institution. In these cases, it’s often positioned as an end, rather than a means.
But there’s a risk to seeing data as an end goal; having data for the sake of it does not always lead to better decision making. There are some instances where it is better to be without data than with it — where the data you’re using is inaccurate, or being incorrectly interpreted, or if it’s not actually used at all in decision making.
It’s often overlooked that collecting and storing data is expensive. And on top of maintenance, you need to contend with the additional burdens of holding data, from updating the privacy policy on your online forms to upgrading your data security.
Given that expense, collecting data should be approached like any product — in iterations and working from a solid, user-first specification. With my product manager hat on, here’s how I’d avoid ending up with an unhelpful, unwieldy set of data.
Make sure the data is needed
A CTO I worked with used to meet any request for more data with: “Will it make you more accurate than your best guess? Does it need to be more accurate than that?” His point was to start out with whether there was actually an issue with taking our best guess — in many cases, this was a valid choice. More data is only of use if it’s likely you will make a different decision, or take a different action, from the one you’re making now.
It’s not just a question of whether more data is needed, but also how much. All I’d offer here is that, while it needs to be decently representative to be of use, you rarely need everything. It’s worth voicing and agreeing with the team what you consider to be representative, and how much margin of error you’re comfortable with. If you do decide to gather it, make sure you’ve made a conscious decision about how long you need it for, too.
The exception to this is if you are working on predictive models, or any machine learning. In this case, go broad and go big.
Make sure the data is accurate
There’s often a sense that data is inherently more accurate than judgement calls. But, as we know, wherever humans are involved — in this case, in the input and analysis — there’s room for error.
If you’re collecting data anywhere under your control, whether it’s your website or your CRM, don’t make it difficult for users to input accurate information. Use human friendly labels to reduce the risk of a user choosing the wrong category or typing in a number in the wrong unit. And banish 47-option dropdowns – it once materialised that our top five reasons for customers choosing a service were not more dominant because they were popular, but because they were the only five the customer success team could remember...
If you’re going to make a field required, you probably need an ‘Other’ option, preferably with a free text addition, to avoid people just picking what’s closest. If you get too many, do a review of the free text and create a new option if it makes a difference in your decision making. Put in a calendar reminder to cleanse your dropdowns every quarter or so – last year, YouTube ads might have been a common response to ‘How did you hear about us?’, but if you’ve stopped advertising there, the option isn’t needed anymore.
Where you have internal staff inputting the information, involve them in what it’s used for. Too often the data is entered, never to be heard of again, and inevitably that decreases staff’s motivation to do so correctly. I’m a big fan of back of the toilet door posters and ad hoc emoji-filled Slack announcements for this — go to where the people are.
Make sure the data is used
As with any product, data is only as useful as how much it’s used. So often, companies undertake huge data collection or visualisation projects only to find that Tableau is only opened once a month for the sales report, and no one can remember the password.
If you’re lucky enough to be starting from scratch, involve non-techies in your data modelling. Words that are logical to a tech team are rarely those which come most intuitively to the rest of the business. I’ve seen hours of work on reports wasted when the results showed that ‘order’, which I’d taken to mean X, actually meant Y. Keep a glossary of the data terms and relations in a format that does not scare non-techies — Github may be great for openness but it’s off-putting for many.
When it comes to third party data visualisation tools, there’s a suite to choose from and often these will be inherited from whatever ecosystem the organisation has invested in. If you do have a choice, I’ve found it’s most successful to use whichever tool the majority are familiar with.
If data isn’t being consumed as readily as you were hoping, or there’s been pushback, avoid swapping visualisation tools as if a new one will be the magic pill. First look at which questions people say the data can’t answer, or what’s taking more time than it should. It may be that a data modelling decision is preventing clear connections — correcting that may be quicker and cheaper than purchasing new software. Before committing to a tool, use the free trial to recreate existing essential reports, answer an outstanding question and combine multiple data sources.
If you do introduce new data visualisation software, it’s not enough to ask business users to ‘have a play around’. No one prioritises this kind of vague instruction. I see it as the responsibility of those introducing the tool to make sure it’s usable and used. I tend to set each data user a specific, real business question to answer (with a deadline), and a task related to someone else’s report. Being able to collaborate on data visualisation and analysis is half the battle.
Is your data actually helping?
Once it’s there, data is often left to age without any checks on its ongoing usefulness or relevance. Use analytics to see if the data visualisation and/or reports are being opened and used. If you’ve introduced a new tool or data set, schedule a check-in six months on to assess usage and examine what more is needed and, as importantly, what can be taken away. Ask yourselves: how has it helped? And what has it changed?
Collecting and manipulating data can be an incredible time sink. I’ve watched months go by while reports are set up, only to see them left unused, or cause more arguments than they resolve due to worries around accuracy. I think it’s because businesses think about data as a one time thing that can be completed and then you ‘have’ it.
But if it can be reframed to a shifting thing that needs planning, iterating and communicating — in other words, thought of as a product that requires product management — the undertaking will be altogether more successful.
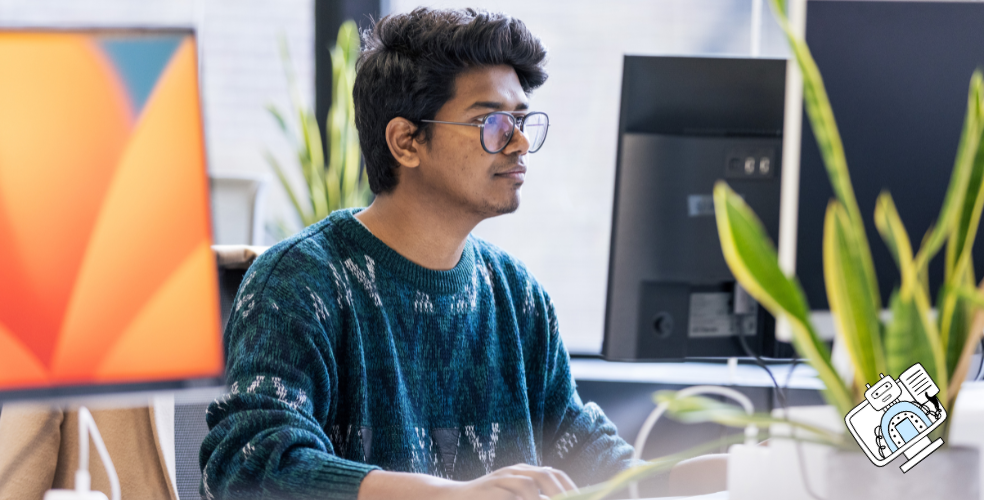
From SQL Server, to Azure, to Fabric: My Microsoft Data and Analytics journey
There is always something new in the Microsoft space, and Fabric is the latest game-changing update to impact the world of analytics.
Read moreOur recent insights
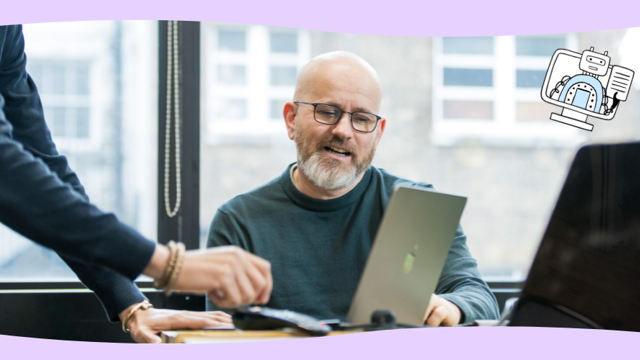
Common misunderstandings about LLMs within Data and Analytics
GenAI and LLMs have their benefits, but understanding their limitations and the importance of people is key to their success.
Read more
Shaping product and service teams
How cultivating product and service teams to support the needs of the entire product lifecycle can ensure brilliant delivery.
Read more
Building ‘The Chatbot’ - Our experience with GenAI
Learn how we harnessed to power of Generative AI to build our very own chatbot.
Read more