The importance of data transparency
If you’re going through all the trouble of collecting data, then hopefully you’re making good use of it.Â
Typical uses of data include gaining a deeper understanding of an organisation or the world in general, deriving insights that help you make better decisions, building new products and services, and improving existing offerings. To do this, data might be analysed, processed, modified, or even shared with people outside of your organisation.Â
Data usage covers a wide scope of activities and throws up numerous ethical issues, particularly around transparency, fairness and bias. This can be particularly problematic when algorithms are used to inform or automate decision-making.Â
The Data Protection Act 2018 says that people who are responsible for using data must make sure that the information is being used fairly, lawfully and transparently. It should also be used for the specified purposes only, as well as in a way that is adequate, relevant and limited to what is absolutely necessary.
Here are our top tips for things to think about to make sure you’re practising data transparency.
1) Be open: Are you transparent about the use of your data?
Data transparency is a fundamental principle in GDPR, and with the increasing use of algorithms in data-driven automated decision-making, it has become even more important to explain how an algorithm arrived at its outcomes.Â
You might have heard many algorithms described as black boxes, in that it’s impossible to know how they really arrive at specific outputs. However, methods to help explain their inner workings (so-called explainable AI) are becoming increasingly used.Â
As well as stating an intended purpose upfront, organisations should also be ready to clearly communicate how the data they collect or acquire is being used. It’s really important to be able to explain how data is driving a decision-making process, or how a particular decision was made from it.Â
Ask yourself:Â
- How open am I about data collection, processing and usage?
- Can I easily articulate and explain how we are using data and for what specific purpose?
- Am I transparent about how we protect and secure the data we collect from consumers or service users?
- If I'm using algorithms and AI, are we using methods to help explain their inner workings and how they arrived at an outcome?
2) Be equitable: Is your data use fair?
To be considered fair, the collection and use of data should be proportionate to the challenge or issue it sets out to resolve. Sometimes it may be difficult to collect data from all groups, but could this lead to unfair outcomes? Â
The quality of data should also be such that the insights it generates are fair and any impact on a particular group can be justified and not considered detrimental to them. It should also be complete enough to drive fair outcomes upon analysis or processing.Â
Ask yourself:Â
- Am I offering customers value in exchange for using their data? For example, is it being used to improve a product or service they use?
- Should I be using personal data at all? Is it fair to do so?
- Am I collecting data in a fair way so that all groups are represented? Are there any gaps?
3) Be honest: Have you acknowledged the existence of bias in your data?
Linked to fairness in data usage is bias. While we acknowledge that humans are often biased and naturally prejudiced, it’s a common misconception that machines make cold and objective decisions. The reality is that they are as susceptible to bias as any human.Â
When you think about it, this makes sense, as whether people are analysing data manually or using algorithms created by humans, processing data can create, reinforce and perpetuate real-world biases. Humans are involved at every stage: generating data, collecting data, making key modelling decisions, and observing and using the outputs. For that reason, human biases are present at every stage, too.Â
When bias is present in your data pipeline, whether through algorithmic bias, data bias, model bias or human bias, it can lead to discrimination. It’s therefore extremely important to be aware of and take steps to mitigate biases wherever possible.
Ask yourself:Â
- Can I identify the points at which biases may have crept into the data, the outputs of analytics and AI, or the human processes using insights to make decisions?
- Are there steps I can take to reduce this bias?
- What level of bias is tolerable? While completely eliminating bias is impossible, how can I strive for the least amount of bias in the system?
- What tools and assistance are available to help minimise bias? Can we explain the decisions our AI models reach?
How predictive policing is entrenching bias within the justice system
There has recently been a growth in the use of predictive analytics within the legal system and law enforcement. Increasingly, AI and machine learning are steering decisions around which neighbourhoods or areas police should focus their attention on (also known as predictive policing).
However, as a report by Durham Constabulary highlights, these algorithms can end up discriminating against certain groups, particularly those from poorer socioeconomic backgrounds. By focusing on particular groups, these AI systems perpetuate biases as target groups become increasingly profiled, gathering more and more data that then reinforces future predictions.
Our tips to reduce bias in your dataÂ
- Review the data, algorithms and human processes involved in decision-making to help identify and assess potential sources of bias. This can go hand-in-hand with implementing ways to measure and mitigate potential biases.
- Consider representation both pre- and post-processing. Are certain underrepresented groups in the data used to train an algorithm?Â
- Are there any inconsistencies or poor predictions for certain demographics or groups in the insights generated?
- The UK Government’s Data and Ethics Framework includes using AI responsibly and defining governance structures.Â
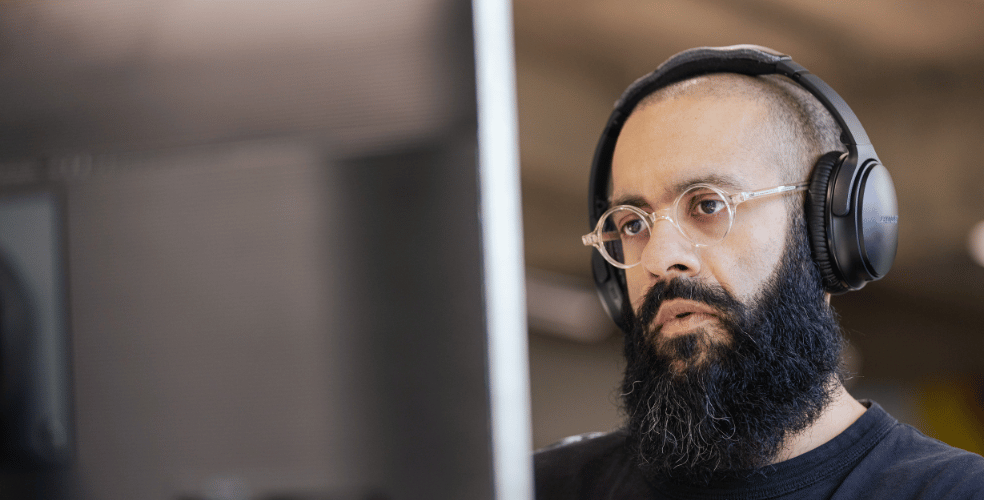
How AI’s reshaping software engineering
AI tools boost dev speed, but not skill. The future is in leading AI with experience, strategy, and thoughtful engineering.
Read more-
3 steps to ethical data collection
Read insight -
A beginner’s guide to data storage ethics
Read insight -
-
The importance of data ethics and how to improve it
Read insight -